Leveraging AI in Decision-Making: From Data Discovery to Decision Support Systems
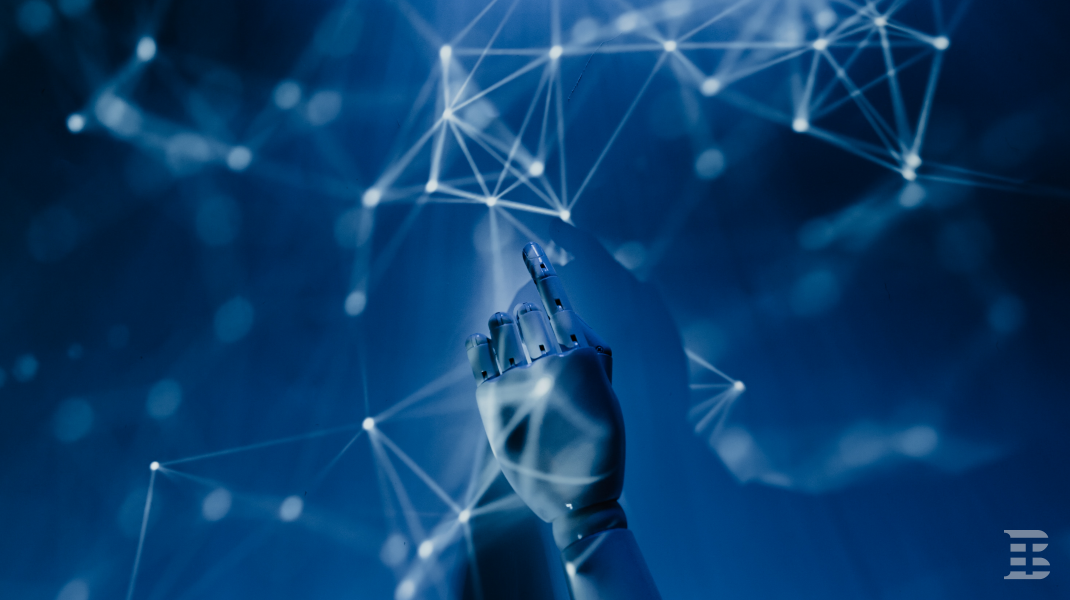
Long gone are the days of gut-based decisions. With more metrics than ever to measure and more data than anyone can ever process, decision-making for businesses can sometimes feel impossible.
Overwhelmed with doubts, data and different stakeholders to please, businesses are turning to AI. It helps them to quickly and accurately analyze large volumes of data, leading the way to the most logical decision – based on numbers, forecasting and algorithms.
But what’s really happening behind the scenes there? Can we just put all business decisions in the hands of an invisible robot?
In this article, we’ll take a peek behind the curtain of AI-based decision-making. We’ll cover:
-
How data is prepared for AI-models
-
How to successfully implement AI in your decision-making process (including the ethical discussion)
-
And how to leverage and level up your AI tools
Ready to make some big decisions, with confidence?
Data Preprocessing and Feature Selection for AI-Based Decision-Making
While marketers and content creators are fearing losing their jobs to ChatGPT, others are understanding a crucial aspect of AI: it’s only as good as the input you give it – and what you end up doing with it.
AI models are dependent on the data they are being fed to be trained. If you want the decisions they help you make to be sensible, you’ll need to know about data preprocessing and feature selection.
Data preprocessing involves cleaning, transforming, and normalizing data to prepare it for AI analysis. It’s done in the data discovery and classification phase, and you can see it as the mise and place a chef does – you’re simply making sure AI models can get to work with the right ingredients.
-
Data cleaning is all about getting rid of inconsistencies and flagging missing values and outliers.
-
Data transformation means you’ll convert all data into a standard format that can be easily understood by the AI model.
-
Normalization involves scaling data to a common range to ensure that no single variable dominates the analysis.
Once your data set is all cleaned up, feature selection comes into play. This involves identifying the most relevant variables or features that will have the greatest impact on the decision-making process.
There are different approaches to identifying the most relevant variables for your specific decision-making dilemma. These feature selection methods can be broadly categorized into three types: filter, wrapper, and embedded methods.
-
Filter methods use statistical tests to evaluate the relationship between each variable and the target variable.
-
Wrapper methods iteratively select subsets of variables and evaluate their performance on the model.
-
Embedded methods involve incorporating feature selection into the AI model itself.
Without these steps and filters, your AI model will work like a blindfolded chef with a blunt knife. Making sure data preprocessing happens correctly is critical in AI-based decision-making, because of the implications a wrong decision can make.
Yes, AI can help make decision-making easier, but accuracy should always come before speed, so don’t trust it blindly if the right protocols have not been deployed.
Implementation and Adoption of AI in Decision-Making
When your business first starts to turn to AI for help in decision-making, it can be scary. Can you trust this technique? What types of decisions can you let AI take care of, and how can you make it fit into your business processes?
Implementing AI in your decision-making processes doesn't happen overnight. To do it the right and safe way, it’s recommended to ease into it using pilot testing and scaling.
Pilot testing
Before throwing high-impact decisions at AI, have it run a pilot project? It can be an imaginary project or one that has already been done. The closer the input is to reality, the better because this is an opportunity to identify potential issues and refine your solution.
Scaling
Once the solution has been thoroughly tested and refined, it can then be scaled to larger test processes. This double layer of testing will help your organization to mitigate most to all risks and ensure that your AI solution is effective before you go all out and invest in a full-scale implementation.
Key ingredients for a successful implementation
Apart from testing and slowly scaling up, there’s more to cover when working towards implementation. Here’s what makes your AI adoption a success:
-
Data governance: to ensure that your data is of high quality, your systems are secure, and you are compliant with relevant regulations.
-
Stakeholder buy-in: it’s essential to ensure that your AI solution is aligned with your organizational goals and that stakeholders are willing to support the adoption of AI.
-
Change management: last but not least, your employees need to be not just willing, but motivated to make the changes that will be brought about by the adoption of AI, including new processes, roles, and responsibilities.
Ethics and AI: an ongoing debate
And a debate that needs to be had, constantly. At the end of the day, we can't actually hold AI accountable for unwanted outcomes, which means businesses need to establish clear guidelines for the use of AI.
When AI is used in decision-making, experts agree it can impact all kinds of human rights concerns. Privacy is an ongoing discussion, bias, and discrimination aren’t eliminated yet, and there are plenty of concerns about safety and security, economic distribution, and even political participation.
Following the most logical path based on data doesn't always lead to an ethical outcome, so oversight and strict rules have to be set in place.
An example of how AI-based decision-making can be more ethical is by actively addressing potential biases in AI.
Biases can be introduced at different stages of the AI process, including data collection, feature selection, and model development.
To mitigate these biases, organizations need to ensure that data is diverse and representative, and that feature selection and model development are conducted in an objective and transparent manner. This simply means that the responsibility and accountability remain with the business.
Leveraging AI in decision support systems
Now you’ve built the foundation, it’s time to build the next level: Decision support systems (DSS), aka the actual tools that will assist decision-makers.
Integrating AI algorithms and human Decision-making
With the right setup, you’ll get the best of both worlds. AI algorithms can process large volumes of data quickly and accurately, providing decision-makers with relevant insights and recommendations.
As accountability, responsibility, and ethics remain important, we’ll never be able to replace human decision-making entirely – nor should we want to. Rather, AI should be used to support and enhance human decision-making by providing data-driven insights and recommendations. And that can be done on more levels than you might think.
Utilizing AI to support decision-making at various levels of an organization
A great thing about AI is its versatility – it can be used to support decision-making at the strategic, tactical, and operational levels of an organization. Here’s what that might look like:
-
At the strategic level, AI can forecast, identify emerging trends and make long-term plans.
-
At the tactical level, AI can help decision-makers make more informed decisions in the short term.
-
At the operational level, AI can be used to automate routine tasks and enable your flesh-and-blood employees to focus on more complex tasks.
Implementing machine learning algorithms and NLP into DSS
Understanding how your AI tool of choice came to its conclusions is crucial, and you can impact its capabilities with more than just the data you feed it.
Machine learning algorithms and natural language processing fine-tune your AI even more.
By incorporating machine learning algorithms, your DSS will be able to analyze historical data to identify patterns and trends, resulting in better decision-making for future situations. And by incorporating natural language processing (NLP), decision-makers can interact with DSS more intuitively, processing queries and providing relevant insights and recommendations.
These "tweaks" are more necessities than nice-to-have features, especially for high-impact decision-making. Plus, it will make your DSS easier to work with, which will give implementation and adoption a big boost.
Example of AI in decision-making
AI is already widely used in decision-making processes for all types of business. Healthcare, financial services, heck – even your Netflix recommendations are all fueled by AI. Here are some examples that show you just how versatile AI is for decision-making:
-
Supply chain management: with data on inventory levels, customer demand, and shipping schedules, companies can optimize their inventory to a tee. For example, a company like Walmart uses AI algorithms to analyze all kinds of sales data and local data to predict demand and optimize inventory levels.
-
Problem-solving and change management: AI can identify the root causes of problems and develop effective solutions by analyzing vast amounts of data – something that would take humans ages. Think about how data is basically the fuel Tesla runs on. They use AI to analyze data from their vehicles, including sensor data and customer feedback, to identify issues and develop targeted solutions.
-
Strategic changes and performance assessment: if you combine past and current data on customer behavior, market trends, and competitor performance, you uncover ways to stay ahead of the competition. Various fast fashion brands are using AI to jump on trends even quicker – and luckily, also helps them become somewhat more sustainable.
-
Customer relationships: we all have experienced the algorithm knowing what we want and need before we even realize it ourselves. Done right, AI can personalize customer experiences and improve customer service. AI can analyze consumers and customers on a deep level, uncovering countless strategic moves businesses can make – from personalized recommendations to specific content.
AI is here to stay, make it worth its while
AI is already revolutionizing countless processes in business, and decision-making won’t be an expectation. With the possibilities that we have today and the knowledge of how to get it right, this is a great time for businesses of all sizes to start exploring the benefits of implementing AI.
Intrigued? Who isn’t? If you want to dive deeper into how AI is affecting specific industries, read on here.
FAQ
Q: Is AI only applicable to large organizations?
A: No, AI can be used by organizations of all sizes. While larger organizations may have more resources to invest in AI development, there are also many affordable AI solutions available for small and medium-sized businesses.
Q: How can AI be used in decision-making without replacing human decision-makers?
A: AI can be used to support and enhance human decision-making without replacing it entirely. By analyzing vast amounts of data and providing insights that were previously impossible to uncover, AI can help human decision-makers make more informed and effective decisions. On top of that, AI can be used to automate routine tasks, allowing human decision-makers to focus on more complex decision-making tasks.
Q: What are some of the challenges of implementing AI in decision-making?
A: One of the biggest challenges of implementing AI in decision-making is data quality and availability. AI algorithms rely on high-quality data to generate accurate insights and recommendations, so organizations need to ensure that their data is clean and properly formatted. Additionally, organizations need to consider the ethical and legal implications of AI-based decision-making, such as bias and privacy concerns.