Understanding Generative Adversarial Networks (GANs) and their Applications
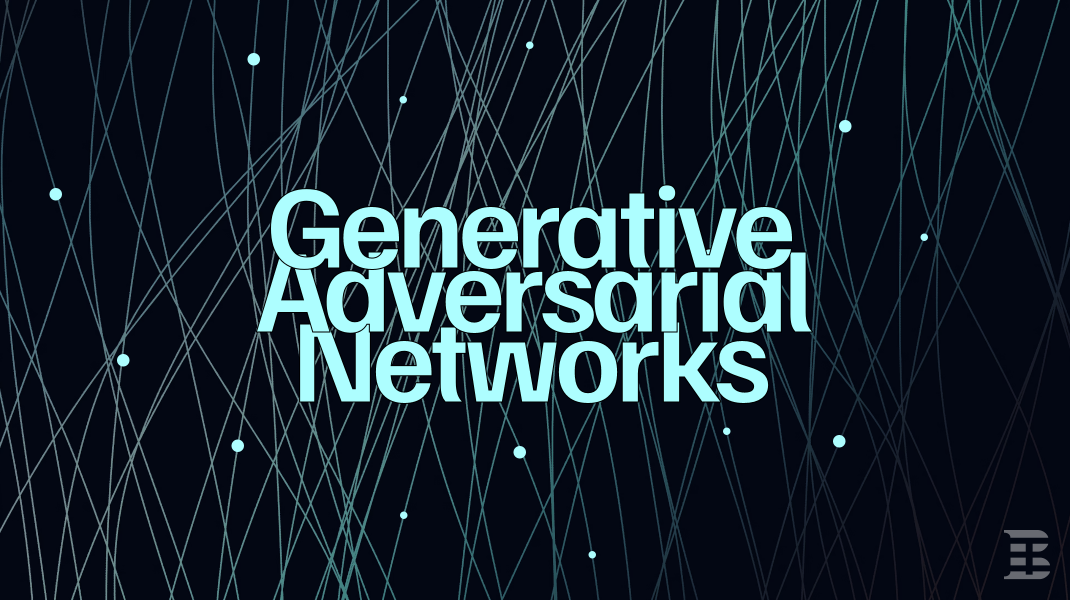
In the ever-evolving landscape of artificial intelligence, Generative Adversarial Networks (GANs) have garnered immense attention and acclaim as a powerful and revolutionary approach. By combining the power of generative models and discriminative models, GANs have unlocked the potential for creative content generation, data augmentation, anomaly detection, and much more.
Developed by Ian Goodfellow and his colleagues in 2014, GANs have transformed the way we perceive and interact with AI systems. This article provides a glimpse into the background of GANs, their fundamental components, and the intriguing adversarial training process that underpins their power.
Background on Generative Adversarial Networks (GANs)
GANs represent a breakthrough innovation in the field of deep learning. At their core, GANs consist of two essential components: a generator network and a discriminator network. The generator network synthesizes new data instances, mimicking the characteristics of a given dataset, while the discriminator network distinguishes between real and generated data. This interplay between the generator and discriminator leads to a fascinating dynamic where the generator strives to produce increasingly realistic outputs, while the discriminator becomes more adept at differentiating between real and fake samples.
During training, the generator and discriminator engage in a competitive game. The generator aims to produce samples that are so convincing that the discriminator is unable to differentiate them from real data. Conversely, the discriminator strives to become more discerning and accurately identify the generated samples. This continuous interplay between the generator and discriminator leads to a convergence where the generator excels at producing authentic-looking outputs.
Applications of GANs
As GANs continue to captivate researchers and practitioners alike, their wide-ranging applications across numerous industries have come to the forefront. From image generation and data augmentation to anomaly detection and beyond, the potential of GANs is boundless. Furthermore, GANs have ventured into music generation, video synthesis, data privacy, and reinforcement learning, opening up new possibilities for creativity and innovation. As GANs continue to advance, their applications are set to expand further, reshaping industries and pushing the boundaries of what is possible with artificial intelligence.
Image Generation and Synthesis: Unleashing Visual Creativity
Generative Adversarial Networks (GANs) have revolutionized the field of image generation and synthesis. Through their remarkable ability to generate realistic and visually appealing images, GANs have found applications in various domains, from computer vision to art and entertainment.
How a generative adversarial network (GAN) works (Molera, 2021).
Imagine an AI system that can produce stunningly lifelike images, indistinguishable from those captured by a camera. GANs make this a reality by learning the intricate patterns and structures of a given dataset and generating novel images that resemble real-world scenes, objects, or people. This capability finds applications in virtual reality, video game design, and even computer-generated movies, where GANs bring imagination to life.
Data Augmentation and Style Transfer: Enhancing Creativity and Design
GANs excel in data augmentation and style transfer, offering a creative twist to the world of fashion, design, and content creation. By leveraging GANs, one can enhance training datasets, generate new samples, and seamlessly transfer artistic styles between images.
In the realm of fashion, GANs empower designers to explore new aesthetics and generate unique clothing designs. By training GANs on existing fashion datasets, designers can generate fresh and innovative styles that push the boundaries of creativity. Moreover, GANs facilitate style transfer, enabling the fusion of different artistic styles onto existing images, leading to visually captivating results.
Anomaly Detection and Novelty Generation: Unveiling the Unusual
GANs have proven to be valuable tools in anomaly detection and novelty generation, with applications in cybersecurity, fraud detection, and anomaly detection across various industries. By learning the underlying patterns of a given dataset, GANs can identify deviations or anomalies that signify potential threats or unusual occurrences.
In the realm of cybersecurity, GANs can detect abnormal network traffic or flag suspicious activities that may indicate malicious intent. By generating novel instances that deviate from the norm, GANs empower fraud detection systems to stay one step ahead of cybercriminals. GANs also find applications in anomaly detection for medical diagnosis, identifying rare diseases or abnormalities in medical images.
Natural Language Processing and Text Generation: A World of Words
By capturing the nuances of language and context, GANs can generate coherent and contextually relevant text, opening up avenues for chatbots, language translation, and content generation. In the realm of chatbots, GANs enable more natural and engaging conversations by generating human-like responses. By training GANs on large-scale conversational datasets, chatbots can mimic human speech patterns and provide more personalized interactions.
Pipelines are commonly used to conduct adversarial-based text generation. (de Rosa, 2021)
Language translation benefits from GANs' ability to generate contextually relevant text. By generating parallel sentences in different languages, GANs aid in the development of more accurate and fluent translation models. Content generation, such as story writing or article generation, can also leverage GANs to produce a compelling and contextually coherent text. GANs empower content creators to explore new storytelling dimensions and generate unique narratives.
Other Applications: Unlocking Creative Frontiers
Beyond the realms of images and text, GANs have been applied to various other domains, offering exciting possibilities for music generation, video synthesis, and reinforcement learning. In music generation, GANs can compose melodies, harmonies, and even entire musical pieces.
By learning from vast musical datasets, GANs generate compositions that resonate with human-like qualities, inspiring new genres and pushing the boundaries of musical creativity. As researchers and practitioners continue to push the boundaries of generative AI, GANs open up new frontiers, revolutionizing industries and reshaping the way we perceive and interact with artificial intelligence.
Challenges and Future Directions
Value in the Generative AI market is projected to reach US $44.89bn in 2023; however, the journey (GANs) is not without challenges and limitations. While GANs have achieved remarkable advancements, several hurdles remain to be addressed. One significant challenge is the instability of GAN training, where the generator and discriminator may reach a stalemate or produce suboptimal results. Additionally, GANs often struggle with mode collapse, where the generator produces limited variations of outputs. Researchers are actively exploring techniques to overcome this limitation, such as using regularization methods or introducing diversity-promoting mechanisms.
In conclusion, the potential impact of GANs across various industries is immense. GANs are poised to revolutionize creative industries, scientific research, and technological advancements, making this an exciting time to delve deeper into the world of GANs.