Five Steps to Start Using AI in Business
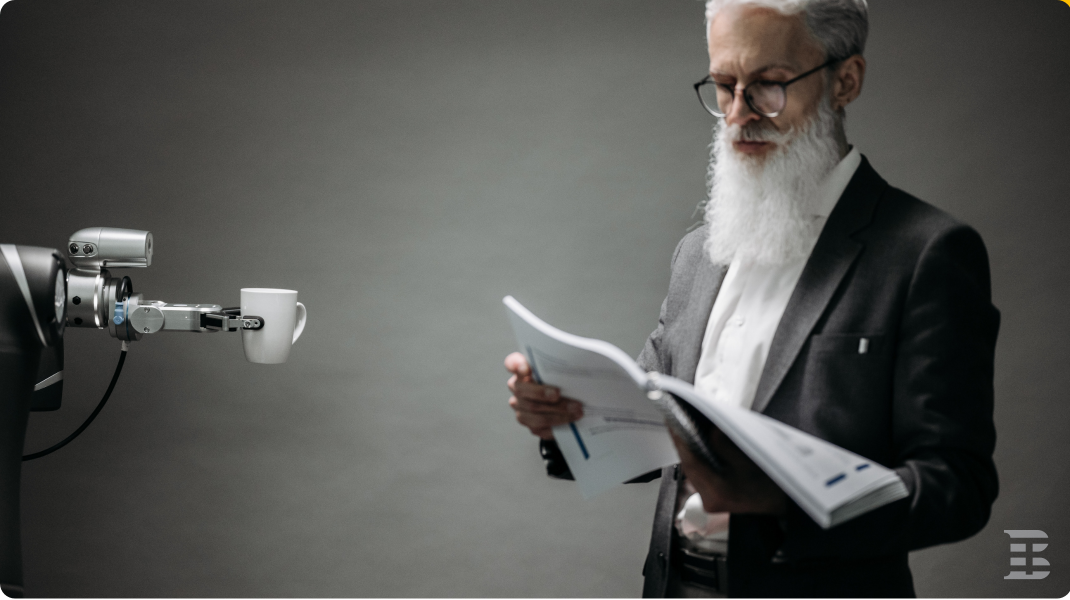
Last year, companies around the globe invested a whopping $93.5 billion in artificial intelligence solutions, up from $67.85 billion in 2020.
This spending spree comes in sharp contrast to the fact that up to 80% of AI projects fail, while just 11% of companies tapping into artificial intelligence see a significant return on their investments.
Does it mean AI is just another buzzword with no actual value behind it?
It’s quite the opposite. The reason for AI projects’ staggering failure rates and lengthy payback periods lies in the fact that few companies know how to implement AI holistically.
A recent survey from Deloitte shows that enterprises with a well-defined AI strategy register a return of over 5% on their AI investments, with an average payback period of 1.2 years. Companies that jump on the AI bandwagon blindly, meanwhile, hardly break even.
So, what do you need to keep in mind before starting using AI in business?
Using AI in Business: 5 Steps to Success
Essentially, we could break down your AI implementation plan into five actionable steps:
-
Get your head around AI and its capabilities
-
Set realistic AI implementation goals
-
Assess your company’s digital maturity and AI readiness
-
Start using AI incrementally with plans for scale
-
Aim for AI excellence
Step 1: Get Your Head Around AI and Its Capabilities
The artificial intelligence term describes machines and software that facilitate or automate tasks, making well-informed decisions the way humans would. To accomplish this, AI needs data, which can be prepared for algorithmic analysis or left as it is.
There are several types of AI that vary in their complexity and cognitive abilities:
-
Supervised machine learning. Such algorithms feed off annotated data, getting excellent at specific tasks — for instance, identifying occupied and vacant spots in a parking lot.
-
Unsupervised machine learning. With unsupervised ML solutions, we could take annotated data out of the algorithm training process, saving software engineers data preparation efforts. Instead, an expert just specifies what type of information databases contain, and algorithms train on their own.
-
Reinforcement learning. When engineers let algorithms roam in the wild without providing instructions regarding how they should process data, we get reinforcement learning. Such AI systems come up with a solution to a problem, and developers can validate the algorithm accuracy. They can further adjust AI performance parameters if the results are unsatisfactory.
-
Deep learning. Powered by deep neural networks, which contain multiple layers of artificial neurons and can therefore evaluate data against various parameters, deep learning solutions have found a permanent home in advanced computer vision (CV) natural language processing (NLP) applications.
Each type of artificial intelligence is best suited for a definitive list of tasks, like interpreting customer sentiment on review websites and or figuring out why your company’s energy bills have soared lately.
By starting using AI in business, however, you will not facilitate all of your tasks and workflows, bringing operational costs to a minimum. In most cases — think running sophisticated industrial equipment or determining whether tumors are benign or malignant — there should be human experts reviewing algorithms’ work and making timely interventions.
Step 2: Set Realistic, Tangible AI Implementation Goals
Before using AI in business, identify the problems you’re aiming to solve with this leading-edge technology — and tie your goals to specific outcomes.
Your AI implementation journey should thus start with a thorough audit of your processes across various departments and job functions. If you can’t do that on your own, enlist the help of experienced technology consultants and business analysts.
In parallel, talk to business units' heads, senior executives, and other internal and external stakeholders. To get a buy-in from the C-Suite, you could also turn to rapid prototyping, creating a proof-of-concept (POC) version of the AI solution.
One thing to add here: your AI objectives should be specific, measurable, achievable, relevant, and time-bound (SMART). For instance, you could deploy NLP-powered chatbots to automate 70% of tasks at your customer support department or handle insurance claims three times faster with intelligent process automation (IPA) tools.
Step 3: Assess Your Company’s AI Readiness
To reach the objectives identified in Step 2, validate that your company has these AI building blocks in place:
-
Technology team. If your organization lacks IT specialists with prior AI development experience, you need to hire tech talent from a third-party AI consulting firm. Another way to start using AI in business is to purchase a no-code or low-code SaaS solution with AI capabilities — but you’ll still probably need to bring in software engineers to set it up.
-
Data. Artificial intelligence algorithms use your company’s data to learn and perform their immediate tasks. This data can be structured (i.e., stored in data warehouses and fully prepared for analysis) or unstructured (i.e., resides in data lakes and lakehouses or external platforms in the form of sensor readings, audio and video files, images, or uneditable documents). Unstructured data comprises roughly 90% of all information an average company generates. To leverage this data for algorithm training and task automation, your company needs proper data storage, retrieval, and analytics infrastructure supported by modern cloud technologies.
-
Computing resources. Speaking of the cloud, vendors like Google, Amazon, and Microsoft offer the resources to train and deploy AI solutions. Therefore, cloud computing becomes key to using AI in business. If your organization still relies on siloed legacy applications that do not support modern tech stacks, you must first create a basis for your AI transformation.
If you’re wondering how to implement AI in a hassle-free way, remember that it’s your employees who can make or break the whole endeavor. With resistance to change being among the leading causes for digital transformation projects’ failure, you must make provisions for employee training and onboarding and addressing ethical challenges imposed by using AI in business.
One way to evaluate the pros and cons of implementing AI in your organization could be conducting the Force Field Analysis, which should be preceded by a thorough assessment of the internal and external factors affecting your operations, as well as the examination of your processes and most valuable assets.
When performing the analysis, assign scores to every factor driving or hindering AI adoption. A positive or negative combined score will indicate whether you’re ready to start using AI in business or whether you need to revamp your IT infrastructure and processes first.
Sample Force Field Analysis. Image source: custom
Step 4: Start Using AI Incrementally with Plans for Scale
The go-to approach for using AI in business is to start small, evaluate algorithm performance, gather user feedback, and scale AI capabilities to other processes and use cases. Learning from your mistakes and transferring that knowledge across your organization is vital, too.
But here comes curious data from Gartner, whose researchers claim only 53% of enterprise AI projects make it from prototypes to production. One reason for the disappointing results could be that most companies approach their artificial intelligence projects as isolated POCs, with no no coherent plans to scale them company-wide.
Therefore, it is important to create a blueprint for your AI implementation plan early on and figure out how the technology will fit into your overall technology strategy. And if a particular AI proof of concept does not deliver on its promise within three months, do not hesitate to pause it and proceed to other use cases.
Step 5: Aim for AI Excellence
Last but not least, how do you measure artificial intelligence project success and validate that it’s worth scaling?
A fail-free strategy to avoid AI implementation mistakes spans:
-
Ensuring effective data management in your organization (for this, you might need to partner with a reliable Big Data consulting company)
-
Interconnecting your applications with the aim of creating a company-wide data ecosystem
-
Establishing an artificial intelligence excellence center led by in-house experts who, with the help of external consultants, will be able to develop new skills, refine algorithm performance, and validate novel ideas
-
Creating a business foundation supporting continuous process optimization and alignment between your organization’s units, decision-makers, and regular employees
A final word of advice: continue experimenting with artificial intelligence even if your initial results are lackluster. Technology has surely been around for decades; however, it’s still in development, getting better by leaps and bounds. And it takes a whole cultural shift to start using AI in business, which means AI pioneers have to undergo both digital and organizational transformation to master the technology and reap its benefits.